Optical Comparators
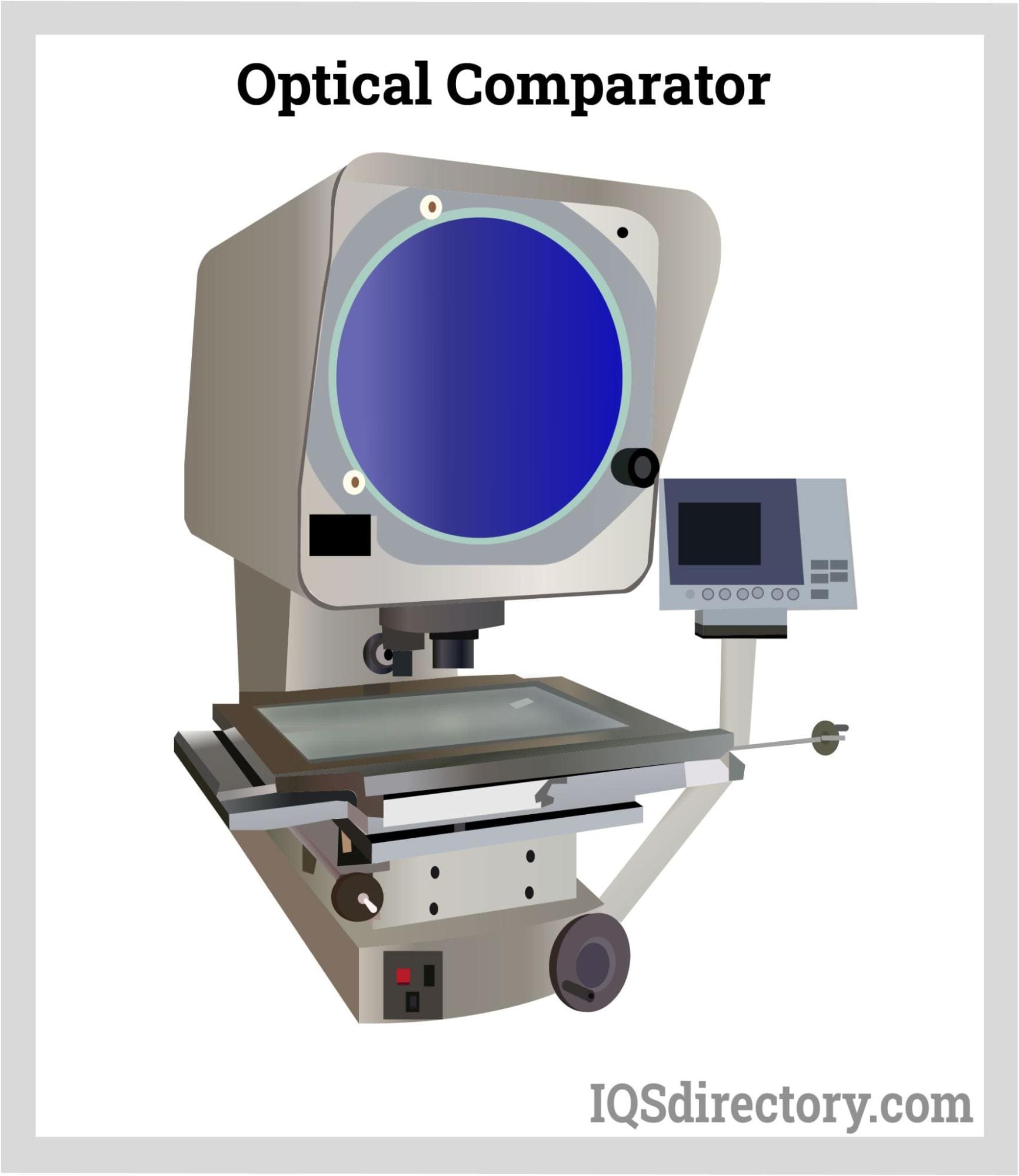
An optical comparator is a measurement system that offers extremely accurate and repeatable measurement data. Optical measuring tools include optical comparators. This gadget employs the principles of optics by utilizing...
Please fill out the following form to submit a Request for Quote to any of the following companies listed on
This article takes an in-depth look at machine vision systems.
Read further and learn more about:
Machine vision systems are made up of interconnected electronic parts, computer hardware, and software algorithms. These elements collaborate to provide operational guidance by processing and examining images captured from the surrounding environment. The information gathered from these vision systems is used for automating and controlling processes or inspecting products and materials.
Numerous manufacturing sectors adopt machine vision systems to undertake tasks that are repetitive, monotonous, laborious, and time-demanding when performed by human workers. This integration results in enhanced productivity and minimized operational expenses. For instance, a machine vision system installed on a production line is capable of inspecting hundreds or even thousands of parts per minute. While human workers could conduct similar inspections by hand, the process would be markedly slower, more costly, and susceptible to errors, besides being constrained by time, which could hinder quality checks on all running components.
Machine vision systems significantly boost product quality and manufacturing yield by delivering precise, consistent, and repeatable detection, verification, and measurement capabilities. They facilitate the early identification of defects during production, thus preventing flawed components from being produced and released into the marketplace. Moreover, these systems enhance traceability and ensure adherence to regulatory standards and specifications in industrial operations.
Machine vision systems are highly specialized automated inspection and imaging solutions found in manufacturing, quality control, robotics, and industrial automation environments. These intelligent systems are typically composed of five core elements, each working in synergy to achieve accurate and high-speed visual analysis. Below, we will explore these critical machine vision system components and their significance in building robust and reliable vision systems. While some elements are familiar from other optical or automation technologies, their integration ensures the advanced functionality that defines modern computer vision, image processing, and automated inspection platforms. Understanding these elements is essential for those evaluating, specifying, or purchasing machine vision solutions for industrial automation or quality assurance tasks.
Lighting is the foundation of accurate machine vision inspection. Proper lighting enables high contrast and clear feature differentiation, allowing cameras and image sensors to detect flaws, defects, or product characteristics with precision. Inadequate lighting results in low-quality images, causing missed defects and unreliable measurements. Key lighting parameters—such as the distance and geometry between the light source, camera, and object; angle of illumination; intensity and color temperature; and the shape and size of the lighting setup—must be methodically optimized. The reflectivity, texture, and material properties of the object surface also heavily influence lighting choices in machine vision applications. Correct lighting set up significantly improves defect detection, alignment accuracy, and part recognition.
Lighting technologies used for machine vision include LED, quartz halogen, fluorescent, and xenon strobe lights, each with distinct spectral, intensity, and energy characteristics. Lighting can be categorized as directional or diffusive, influencing how features and defects are brought into focus. Optimal lighting reduces errors, increases inspection line speeds, and ensures image consistency—vital to machine vision applications like optical character recognition, barcode reading, parts presence verification, and surface quality inspection.
Backlighting involves placing the light source behind the target object. This illumination technique creates strong contrast—objects appear as dark silhouettes against a bright field—making it highly effective for identifying holes, gaps, foreign material, cracks, bubbles, or scratches in transparent or translucent parts. Backlighting is widely used in dimensional measurement, gauging, sorting, positioning, and edge detection, especially for vision-guided robotics and automated assembly. For the highest accuracy, monochrome light and polarizers are used to suppress glare and enhance edge definition.
Diffuse (full bright field) lighting spreads light in multiple directions, ensuring uniform, shadow-free illumination. This is particularly effective for shiny, textured, polished, or mixed reflective surfaces, as it minimizes glare and prevents specular highlights from overpowering the image. Three primary types of diffuse lighting are used in machine vision:
Partial bright field or directional lighting uses light beams directed from specific angles to accentuate surface texture, edges, and elevation changes, providing excellent contrast for inspecting topographical features such as ridges, depressions, and markings. However, this method may yield unwanted reflections with highly reflective (specular) materials, potentially creating hotspots in the camera image. It is effective in surface flaw detection, printed circuit inspection, and verification of embossed or stamped features.
Dark field lighting features low-angle illumination, where light strikes the object nearly parallel to its surface, revealing small surface defects by causing them to scatter light toward the camera. This lighting approach makes scratches, pits, imprints, notches, dust, and other fine anomalies appear bright against an otherwise dark field. Dark field lighting is frequently employed in glass inspection, metal surface analysis, medical device manufacturing, and precision metrology.
Specialty lighting accessories—such as color filters and polarizers—are often integrated in machine vision lighting systems to fine-tune image quality. Color filters adjust the contrast of certain features or isolate specific wavelengths to highlight desired areas, while polarizers help eliminate unwanted reflections and glare, further enhancing the sharpness, accuracy, and repeatability of automated inspections in challenging lighting environments.
The lens is a critical component of machine vision cameras, responsible for capturing and focusing light onto the image sensor. The choice of lens significantly impacts image clarity, resolution, and the ability to detect minute features. Lenses designed for machine vision often provide color correction or chromatic aberration minimization for enhanced image fidelity. There are interchangeable options—such as C-mount and CS-mount lenses—that offer flexibility for different inspection distances and fields of view, as well as fixed-focus lenses for dedicated applications. Proper lens selection is fundamental to successful industrial vision, measurement automation, and quality control tasks.
The image sensor is the heart of any vision camera, transforming optical images into digital signals for subsequent processing. Modern machine vision cameras use either CCD (charged-coupled device) or CMOS (complementary metal-oxide-semiconductor) sensors, each offering distinct advantages for certain inspection environments. CCD sensors typically deliver lower noise and higher image uniformity, while CMOS sensors offer faster frame rates and greater integration flexibility—both are staples in today's high-precision automated visual inspection systems.
Two main specifications govern image sensor performance: resolution and sensitivity. Resolution refers to the number of pixels composing the digital image; higher-resolution sensors enable detection of smaller features, critical for electronics inspection, pharmaceutical packaging, and semiconductor manufacturing. Sensitivity measures the sensor’s ability to register minimal light levels, ensuring reliable detection even under low-contrast or high-speed scenarios. Striking the right balance between high resolution and optimal sensitivity is key for superior inspection results across varying application requirements.
Additional considerations when selecting an image sensor for a machine vision system include dynamic range, noise performance, and frame speed, which can affect throughput in high-speed production lines and industrial robotics.
The vision processing unit (VPU) is responsible for executing image processing algorithms, transforming raw digital images into actionable information. Using sophisticated software and artificial intelligence algorithms—such as pattern recognition, geometric search, defect analysis, and optical character recognition—the VPU deciphers complex scenes and delivers high-speed, high-accuracy automated inspections. Processing can occur within embedded smart cameras or on external industrial PCs, depending on system architecture and processing requirement.
Common vision software functions include image enhancement, edge detection, morphological analysis, measurement, feature extraction, defect classification, and pass/fail decision-making. For modern quality assurance in manufacturing, such as automotive, electronics, and food processing, the vision processor often integrates with data analytics, machine learning, and traceability systems, providing valuable process insights and supporting predictive maintenance.
The communication system enables seamless data exchange between the vision processing unit and the rest of the automation environment. Fast and reliable input/output (I/O) interfaces—such as discrete signals, PLC connections, RS-232 serial communication, Ethernet/IP, Profinet, and industrial fieldbus standards—are crucial for integrating vision systems into automated machinery, robotics, and control systems. High-speed communication ensures immediate transfer of inspection results, triggering appropriate downstream actions, such as rejecting defective parts, sorting products, adjusting robotic movements, or updating MES/ERP databases for traceability.
For end users evaluating machine vision solutions, compatibility with existing production line equipment, straightforward integration capabilities, scalable networking, and cyber-security protections are essential considerations when specifying vision system communication hardware and protocols.
There are several main categories of machine vision cameras used in industrial automation, quality control, and automated inspection systems. Choosing the right type of camera is crucial for optimizing image acquisition, improving inspection accuracy, and enhancing manufacturing efficiency. Here are the primary types of machine vision cameras:
A line scan camera is an essential component for high-speed industrial inspection applications. It captures images in a sequential manner, scanning one line of pixels at a time. While the camera does view the entire object, the complete digital image is constructed within software by assembling individual pixel lines as either the camera or the object moves. This scanning technique enables the inspection of items moving rapidly along a production line.
Line scan cameras are particularly effective for inspecting continuous materials like paper, metal sheets, plastics, textiles, and web-based products, as well as for examining cylindrical or elongated objects. Their ability to deliver seamless, distortion-free images makes them ideal for conveyor belt systems, print inspection, bar code reading, and process monitoring in the food & beverage, pharmaceutical, and packaging industries.
Area scan cameras use rectangular image sensors to capture images in one complete frame, generating a full field-of-view snapshot. The resulting digital image reflects the pixel dimensions of the sensor, making it easy for the vision processing unit to analyze individual scenes. Area scan cameras are highly versatile and are among the most widely used in industrial machine vision setups. Their straightforward installation, calibration, and alignment make them user-friendly, and they excel at inspecting stationary objects or items that can pause briefly on the production line.
These cameras are ideal for defect detection, part identification, quality assurance, barcode reading, surface inspection, and electronic component testing. They work seamlessly with both monochrome and color imaging and are compatible with integrated machine vision software, making them suitable for a variety of industries including electronics, automotive, medical device manufacturing, and semiconductor fabrication.
3D scan cameras deliver advanced capabilities for in-depth inspection, measurement, and object recognition. They capture image data across the X, Y, and Z planes, determining position, spatial orientation, and volumetric attributes of objects. These cameras utilize either single or multiple units paired with laser displacement sensors or structured light to generate depth information.
In a single-camera setup, the camera produces a detailed height map by measuring the displacement of a laser on the target, enabling precise calculations of object height, surface topography, and flatness. Multi-camera systems utilize laser triangulation to create comprehensive 3D profiles, supporting tasks such as robotic guidance, bin picking, and inline metrology.
With robust performance in challenging environments—including varying lighting, contrast, and color conditions—3D scan cameras are indispensable in factory automation, part orientation, defect analysis, and reverse engineering. They play a crucial role in advanced metrology, smart manufacturing, and machine learning-based inspection solutions.
It may be presumed that optical comparators and machine vision systems are the same type of technology and perform identical functions. While both solutions are designed for part inspection and quality assurance, there are fundamental differences in their methodologies and capabilities within industrial processes.
Both optical comparators and machine vision systems are employed to evaluate, measure, and verify the dimensions and parameters of manufactured parts. However, optical comparators are limited to two-dimensional (2D) or two and a half dimensional (2½D) assessments of small components without software integration. They function by projecting a silhouette or profile view, lacking the advanced digital image processing, automation, and integration features required for modern manufacturing. Optical comparators also do not connect to computer-aided design (CAD) files or automated data analysis tools.
Because optical comparators require manual operation—wherein the operator positions each part individually—they can be slow and labor-intensive, restricting throughput and limiting their suitability for high-volume production environments. They have fundamental design limitations and are unable to match the speed, repeatability, process automation, and tight tolerances achieved by advanced machine vision systems.
Despite improvements in optics and lighting over the years, the core technology of optical comparators has changed very little since their introduction in the 1920s. In contrast, machine vision systems offer automated measurement, defect detection, part identification, 3D capabilities, and seamless integration with robotic systems and manufacturing execution software. With support for complex CAD-based inspections, automated metrology, image analysis, and real-time quality control, machine vision solutions are rapidly replacing traditional comparator technology in demanding production settings.
When selecting between optical comparators and machine vision cameras, manufacturers should consider key factors such as required inspection accuracy, production speed, automation level, data traceability, and integration with quality management systems. For most advanced industrial inspection tasks, machine vision cameras provide superior flexibility, reliability, and long-term scalability in challenging and dynamic manufacturing environments.
Hyperspectral imaging expands upon traditional spectral imaging by utilizing a broader range of wavelengths to capture data from each pixel individually. Unlike standard spectral imaging, which typically captures only a few colors such as red, green, blue, and near-infrared, hyperspectral imaging can analyze hundreds of distinct wavelengths. This advanced capability allows hyperspectral machine vision systems to identify internal variations and impurities within an object, beyond just surface-level defects.
The increasing adoption of hyperspectral imaging systems can be attributed to their ability to deliver rapid and precise data. They have become crucial in sorting processes due to their efficiency and high-quality, error-free control. Hyperspectral imaging excels at differentiating between substances with subtle chemical differences, such as various types of plastics, even when they appear visually similar. This technique is particularly effective for analyzing opaque materials that do not allow visible light to pass through.
Hyperspectral imaging systems offer inspection capabilities beyond the reach of conventional cameras that focus solely on surface analysis. This sophisticated technology is expected to increasingly become the benchmark for quality control in various industries.
Industries that incorporate hyperspectral imaging into their machine vision systems include:
While pills in a batch may appear uniform to both cameras and human observers, hidden impurities and defects beneath their surfaces can go unnoticed. Hyperspectral imaging is capable of identifying and marking these issues for removal.
In food production, hyperspectral machine vision systems can identify contaminants like maggots and non-food objects such as rocks or branches in vegetable batches. They are also useful for detecting impurities or contamination in processed foods like cheese or sausage.
Hyperspectral imaging is effective in spotting impurities, damp areas, knotholes, or resin pockets in wood used for construction.
In the medical field, hyperspectral imaging helps in detecting and identifying hidden cancer cells.
Hyperspectral cameras decompose a signal into its spectral components, projecting each component onto a single pixel with minimal energy exposure. The technology behind hyperspectral imaging is evolving rapidly, extending its spectral range from the usual 930 to 1700 nm to a broader range of 1700 to 2500 nm, accommodating a variety of materials.
Machine vision systems offer cutting-edge and efficient solutions by automating tasks frequently carried out in industrial processes:
Presence inspection involves verifying the number and existence or non-existence of parts. It is a fundamental function of machine vision systems and one of the most common tasks across various industries. Typical applications include counting items like bottles and screws, verifying label presence on food packages, checking electronic components on PCBs, ensuring proper adhesive application, and confirming the presence of screws or washers in assembled parts.
Machine vision systems utilize the following image processing techniques:
Binary processing involves converting images from a monochrome camera into pixels with only two shades: black and white. This simplification facilitates easier vision processing and decision-making. Each pixel is converted based on a predetermined threshold value.
After binary processing, the resulting digitized image undergoes Blob analysis. A "blob" in this context refers to a cluster of pixels with identical shades. The image is mapped onto a coordinate system, where the X and Y positions of each blob are identified and assessed.
Blob analysis is employed for various tasks, including counting based on the blob’s area, measuring dimensions and areas, pinpointing the location of objects, determining the orientation of items, and detecting defects, among other applications.
Additional techniques for image processing and analysis include:
Positioning is the process of comparing the location and orientation of the part to a specified spatial tolerance. The location and orientation of the part in 2D or 3D space are communicated to a robot or a machine element for it to align or place the target in its proper position or orientation. Machine vision positioning systems offer more accuracy and speed than manual inspection, alignment, and positioning. Practical positioning applications include robotic pick-up and placement of parts on and off the conveyor belt, positioning of glass substrates, checking of barcode and label alignment, checking of IC placement in PCB, and arrangement of parts packed in a pallet.
Machine vision identification involves scanning and interpreting barcodes, 2D codes, direct part markings, and text on products, labels, and packages. These codes can include details such as product name, manufacturer, date of manufacture, lot number, and expiration date. This process enhances traceability, inventory management, and product verification. Identification is achieved through optical character recognition (OCR) or optical character verification (OCV) systems. OCR systems interpret printed alphanumeric characters on the target without pre-set character data. In contrast, OCV systems confirm the presence of specific character strings.
Flaw detection is a crucial aspect of quality control in manufacturing and a primary application of machine vision systems. This process involves identifying defects such as cracks, scratches, blemishes, gaps, contaminants, discoloration, and other surface irregularities that may impact the product's performance and reliability. Defects often appear randomly, so machine vision algorithms are designed to detect changes in patterns, color, texture, or structural continuity. The system monitors for these defects and can classify them based on type, color, texture, and size, enabling the segregation of faulty parts. Machine vision systems excel at detecting minute and microscopic defects that may not be visible to the human eye and can operate continuously over extended periods, unlike human inspectors.
Flaw detection is widely used to inspect semiconductor and electronic components, appliances, tooling conditions, food products and their packaging, materials produced in continuous webs (e.g., paper, plastics, metals), and others. Flaw detection is useful in online inspections; once a failing part is detected coming from a process, the process is halted immediately and corrected, and the failing part is separated from its lot. Flaw detection is typically incorporated in machine vision systems together with presence inspection, measurement, and positioning functions.
Measurement involves assessing the dimensional accuracy and geometric tolerances of parts. Machine vision systems calculate distances between multiple points and the positions of specific features on an object to verify whether they meet specified standards. Achieving precise, accurate, and consistent measurements requires optimizing both the lighting and optical systems within the machine vision setup.
Machine vision systems are capable of measuring features as small as 25.4 microns. This functionality is often combined with flaw detection to evaluate the irregularities found in parts. Additionally, these systems can be used to determine the volume of components.
Machine vision systems have a wide range of practical uses across various industries. Some of the key industry-specific applications include:
Ensuring accurate packaging and identification of food, beverages, pharmaceuticals, and consumer products necessitates a dependable and resilient inspection system.
In semiconductor quality control, detecting flaws and accurately positioning components are essential tasks. Machine vision systems play a crucial role in addressing these needs effectively within the industry.
Designing a machine vision system involves the complex task of integrating diverse and distinct components into a cohesive, efficient unit. The first stage includes defining the system's functions and its operational methods.
Every facet of the design process is meticulously assessed to ensure that all machine vision elements, components, and principles align with the specific goals and requirements of the application.
The term "camera" refers to the component responsible for capturing images in a machine vision system. Key requirements include detecting features, identifying objects, pinpointing locations, measuring dimensions, and processing speed. After defining these requirements, you can then specify the necessary spatial resolution, image resolution, and frame rate for the application.
The spatial resolution refers to the number of pixels of the smallest feature to be processed or the precision and repeatability that must be met. Very small features, such as holes or bolts, require very few pixels but resolution will not be reliable. To improve resolution, more pixels will be necessary to improve spatial resolution.
In measurement applications, pixel fractions can be employed, with a minimum precision of one-tenth of a pixel. The acceptable pixel size or fractions are based on the measurement accuracy required.
When evaluating size and measurement, it's crucial to consider both types of spatial resolution, with the finer resolution generally being preferable.
Image resolution is determined by the number of columns and rows required to achieve the desired spatial resolution. To calculate image resolution, divide the image area by the spatial resolution to find the necessary pixel count, width, and height. The camera should have more rows and columns than this calculated value.
The last step in choosing a camera is to decide the required frames per second for the application. Most machine vision systems run at 10 to 15 frames per second or less. Higher resolutions will generally result in slower image rates.
Selecting a lens involves considering its format, field of view, distance to components, and optical resolution. Calculations required include the lens’s optical resolution, magnification, and focal length.
Lenses are designed for specific sensor sizes and must match the sensor’s size. Ensuring compatibility between the lens format and the sensor format is crucial. Lens mounting is influenced by the camera and sensor dimensions, with C mounts suitable for low to medium resolution sensors.
Optical resolution describes the lens's capacity to distinguish between various feature sizes, from small to large.
Magnification is calculated by dividing the smallest measurement of the field of view by the smallest sensor dimensions. This factor is influenced by the lens’s focal length and the working distance.
The light source in a machine vision system is crucial for creating contrast between the component and its background. Accurate calculations are necessary due to the wide range of available lighting techniques.
After selecting the camera, lens, and light source, it's crucial to test them to verify they meet the required performance specifications. Actual application tools should be used during testing.
Evaluation references should include:
Testing the machine vision system might reveal the need to modify or replace components to more effectively achieve the application's objectives.
An optical comparator is a measurement system that offers extremely accurate and repeatable measurement data. Optical measuring tools include optical comparators. This gadget employs the principles of optics by utilizing...
An automatic screwdriver is a piece of equipment that automatically inserts screws into a product during assembly and production. Since every production operation is unique and requires a...
An automation system is an integration of sensors, controls, and actuators designed to perform a function with minimal or no human intervention. The field concerned in this subject is called Mechatronics which is an...
A bowl feeder is a mechanism for supplying small parts and components to a production line or for sorting bulk items for rapid use. A self contained bowl feeder system has a bowl that sets on a spring loaded base that moves vertically...
A conveyor system is a method for moving packages, products, supplies, parts, and equipment for production, shipping, or relocation. The different types of conveying systems include pneumatic, screw, belt, and roller. The construction of individual systems depends on the materials...
Collaborative robots, also abbreviated as Cobots, are the newest technology in robotics. They have changed the automation world significantly. These robots can work safely together with workers, hence are...
An industrial robot is an autonomous system of sensors, controllers, and actuators that executes specific functions and operations in a manufacturing or processing line. They operate continuously through repetitive...
Labels are an important aspect of product packaging, identification, presentation, and traceability. They are a way of communicating the manufacturer to the customers and the rest of the world. Labels promote the brand of the product and...
Packaging equipment is utilized throughout all packaging processes, concerning primary packs to distribution packages. This involves many packaging operations: cleaning, fabrication, filling, sealing, labeling...
Robotic system integrators are companies that provide assistance in automating a wide range of applications. They help design robotic technologies that best suit the needs of an operation for a company and solutions as...
Vibratory conveyors are material-handling equipment used to transport fine to coarse-grained bulk materials. These vibratory conveyors are strong conveying equipment utilized for bulk commodities with fine to coarse graininess...
Vibratory feeders are short conveyors used to transport bulk materials utilizing a controlled vibratory force system and gravity. The vibrations impart a combination of horizontal and vertical acceleration through tossing, hopping, or sliding-type of action to the materials being handled...
A vibratory screening is a process that separates bulk solid materials from solids and slurries using inertial vibration that causes various sizes of particles to pass through openings in a screen or...
Warehouse automation is the process of replacing repetitive tasks with systems that are automated. The main goal is to remove labor-intensive duties that consume time. As a result, the workers can focus more on...